Anti-Patterns in Tech Cost Management: No/Bad Metrics
Series Index
Introduction
Anti-Pattern 1: Not considering scale
Anti-Pattern 2: Bad cloud strategy
Anti-Pattern 3: Inability to assign/attribute costs
Anti-Pattern 4: No metrics or bad metrics
Anti-Pattern 5: Not designing it in
Anti-Pattern 6: Cost management as a standalone
Anti-Pattern 7/8: No ongoing reviews (current and potential)
Anti-Pattern 9: Across the board cuts
Anti-Pattern 10: “A tool will solve the problem!”
Anti-Pattern Bonus: Don’t do rewards programs!
A few thoughts: Three things you can do right now, for yourself and your team
Wrap up
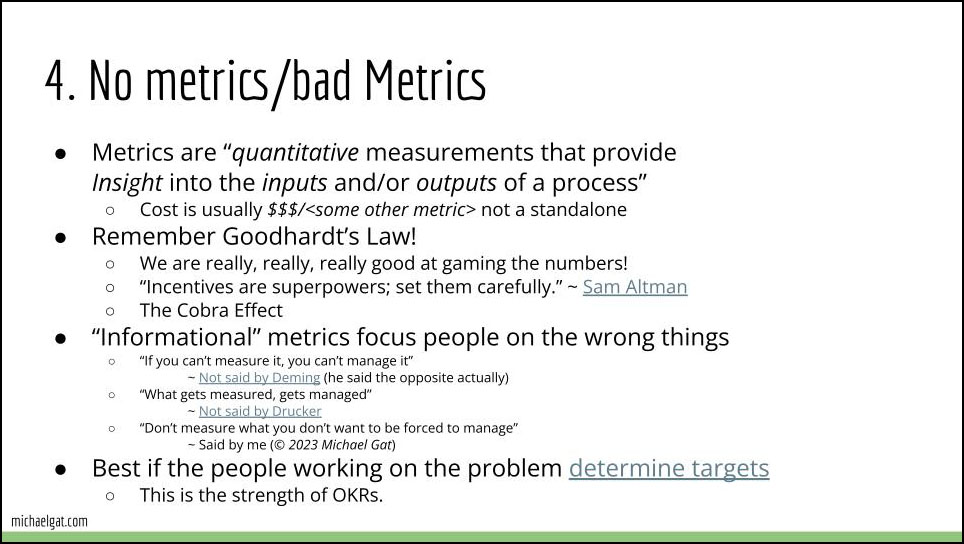
This is one of the most important points, and one that I see frequently violated. Managing costs is not possible if you don’t have good costs metrics in place. The topic of how to create good cost metrics is worthy of a full talk (or book) in itself.
The best short definition of “metrics” I have seen is the one included in the slide. Metrics are quantitative measurements that provide insight into the inputs and/or outputs of a process.
- They are quantitative. There is definitely room for qualitative evaluation of everything we do, but metrics are quantitative, which among other things means they can be tracked over time and compared across different business units, applications, teams, etc.1
- They provide insight. One key mistake is to assume that a metric provides a clear cut description of what the problem is, how to solve it, or even whether there is a problem at all. But good metrics provide insight into all these questions, that allow for further investigation.
- They deal with the inputs and outputs of a process. In cost management, cost is typically an input. Whatever you manage to do with the money spent is an output. While the input cost on it’s own can be useful, especially when broken down and attributed appropriately (see the previous anti-pattern), many of our metrics are cost/<some output>. I can reduce your cloud bill to zero if the outputs don’t matter.
An example of this was my first role at AWS. I was responsible for estimating and ensuring the availability of both virtual and physical infrastructure required for one of our networking services. The annual budget when I left was over $70m. It had more than doubled in the time I was there. Yet, I claim to have saved approximately $42m in the time I was there. How? Because had we continued to spend as much per terabit of network traffic as we had when I started, that’s how much more we would have spent over those 3 years. Looking at the budget in isolation, I was spending a lot more. Looking at it as a relationship, I saved a lot.
Goodhardt’s Law
“When a measure becomes a target, it ceases to be a good measure.”
or more precisely, as stated by Goodhardt:
“Any observed statistical regularity will tend to collapse once pressure is placed upon it for control purposes.”
It’s a subset of the more generalized observer effect that you may be familiar with from various fields.
As engineers, we are experts at gaming the numbers. Once we know what the target is, we will optimize our activities to hit the target, not necessarily to do the right thing. There will be several examples of this in the coming slides. So it’s important that the incentives be aligned with the long-term behavior required.
Strongly consider perverse incentives and “the Cobra Effect.”
Don’t measure it if you don’t want to manage it
We should be especially careful about collecting too many “informational” metrics. Nobody believes that the data is purely “informational” and once it’s collected, it will be acted on. You should be wary of creating bad metrics by collecting “informational” data that you don’t have specific plans for use. (An exception could be a data warehouse or data lake where your are collecting a lot of data, with no specific metrics calculated or implied.)
- “If you can’t measure it, you can’t manage it”
~ Not said by Deming (he said the opposite actually) - “What gets measured, gets managed”
~ Not said by Drucker - “Don’t measure what you don’t want to be forced to manage”
~ Said by me (© 2023 Michael Gat)
Much has been written about this effect. The Thomas theorem is a theory of sociology which was formulated in 1928 by William and Dorothy Thomas:
“If men define situations as real, they are real in their consequences”
V. F. Ridgway wrote in 1956 that:
“Even where performance measures are instituted purely for purposes of information, they are probably interpreted as definitions of the important aspects of that job or activity and hence have important implications for the motivation of behavior”
Ridgway also inspired the quote often attributed to Drucker, which was written by reviewer Simon Caulkin about his work. Ridgway’s writings are nuanced and critical. He recognizes that there is a wide chasm between “what is managed” and “what people will be motivated to focus on.” Most importantly he recognizes that just the perception that something is being managed will motivate people, regardless of the management reality. The full quote, that means something very different from the thought attributed to Drucker is:
“What gets measured gets managed – even when it’s pointless to measure and manage it, and even if it harms the purpose of the organization to do so.”
Who owns the metrics?
Finally, be careful about who creates the targets for your metrics. The strength of the OKR approach is that ideally, senior management sets up objectives and those “in the trenches” suggest key results (metrics) to measure progress towards those objectives. This process breaks down and becomes unmanageable when either:
- Senior management set the KRs without consulting the individual teams doing the work (I will have more on this later)
- Some third-party sets the KRs (or individual tasks), at scale, across an organization, without understanding how they impact individual teams. For example, when somebody decides “all teams must…”
I have never seen a successful large-scale program succeed when the scope and measures of success for the work required was determined by somebody other than the people who were intimately familiar with it. This is true for cost programs as well as all other efforts.
Key takeaway
I could continue to cite people far smarter than me for a long time. This is a very rich area and ties into the forecasting space that I’ve intentionally excluded. As I said at the top, addressing how to go about metrics could be a presentation in itself, and likely a series of them. But for the purpose of this anti-pattern, make sure you have metrics, that they are relevant and that you’ve thought about any perverse incentives you may be creating.
- The argument about whether to use qualitative or quantitative measurements and what the benefits and drawbacks of each are, goes all the way back to Aristotle, possibly earlier. ↩︎
Join us at the SoCal Linux Expo (SCaLE 21x) in Pasadena on March 14-17. My talk will be on Saturday the 16th. I will also be speaking at UpSCaLE on Friday night, and running the Observability track on Saturday and Sunday.
It’s $90 for four days of great content. (If you know me, ping me as I may have a few discount passes left.)